Dr. Sharifa Alghowinem: A Journey with Social Robots: Opportunities, Challenges, and the Future
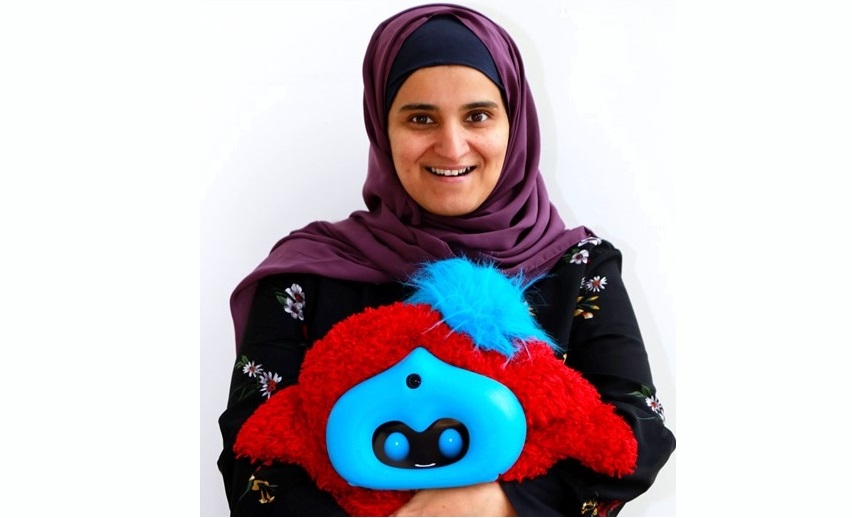
In this webinar, Dr. Alghowinem will walk you through her journey with the social robots. Dr. Alghowinem worked with Prof. Cynthia Breazeal's Personal Robots Group at MIT's Media Lab to design robots to be compassionate companions and knowledgeable assistants to individuals and families. Their robots were deployed to people's homes to coach them in positive psychology, where they help to improve individuals' moods. Integrating the robot with external sensors, the robot is able to assist on several health aspects, such as medication adherence. Moreover, in the family activities context, the robots were able to interact during the story-reading activity to induce conversation and encourage asking questions about the story, to help the child in critical thinking. Besides linguistic understanding, AI and modeling for verbal and nonverbal behaviors, are the main fields in equipping the robot with the ability to understand social cues. In this webinar, Dr. Alghowinem will also connect the projects with the AI modeling associated with them. This will provide a full overview of the opportunities and challenges, as well as how we move forward for the future.
WEBINAR TRANSCRIPT
DOROTHY HANNA: Good afternoon everyone and good evening to anyone joining us from Saudi Arabia. My name is Dorothy Hanna and I'm the program administrator for the KASCST event, Khaldun Fellowship for Saudi Arabian Women here at MIT. Welcome to today's IBK webinar, which is presented by Dr. Sharifa Alghowinem. And it's based on her research here at MIT this past year and more, which is titled a journey with social robots, opportunities, challenges, and the future.
So if you have questions, please post them in the Q&A feature as we go along, and we will get Dr. Sharifa to answer them for us at the end. And before Dr. Sharifa gets started, our program director professor Kamal Youcef-Toumi will introduce Dr. Sharifa as advisor, professor Cynthia Breazeal.
KAMAL YOUCEF-TOUMI: Great. Thank you, Dorothy. Thank you. It's an honor to introduce professor Cynthia Breazeal. She's a professor of media arts and sciences at MIT. She founded and directs the Personal Robots Group at the Media Lab. She's also associate director for The Bridge, this is MIT'S quest for intelligence, where she leads several strategic initiatives in different areas, including democratizing AI through K to 12, and also vocational education.
Cynthia also founded the consumer social robotics company, Jibo, where she served as a chief scientist and the chief experience officer. And also in addition to her works, she has a seminal book, Designing Social Robots. This book is recognized as a landmark in launching the field of social robotics and human robot interactions. Her recent work focuses on the theme of living with AI, and understanding the long term impact of social robots that can build relationships and provide personalized support as helpful companions in daily life.
So thank you, Cynthia for being with us today. And on behalf of the program, we thank you for not only hosting Sharifa but also supervising her work and working with her closely in your lab. And perhaps I'll ask you, Cynthia to say maybe a few words about Sharifa before she makes her presentation.
CYNTHIA BREAZEAL: Yeah, absolutely. So it's wonderful to be here and to hear Sharifa talk about the work that she's done in my group for the past year or so. Sharifa has been very prolific. She's actually worked on a number of different projects. So all of them have this theme of using AI and machine learning to understand human behavior and human interaction, whether that's between people or with a robot, or just understanding these really important subtle social cues that can give signals to things even things like mental health and wellness.
So the algorithms and the work I think are really significant and important beyond social robots, although social robots is kind of the context that we study this work within my lab. She's been publishing on this work. So again, has made a number of really nice contributions, I think, to the field.
KAMAL YOUCEF-TOUMI: Great. Yeah. Thank you. Thank you for the great introduction. Sharifa, the floor is yours.
SHARIFA ALGHOWINEM: Yap. Thank you, Cynthia for the introduction and kind words. I've had a great environment and very productive. So I wouldn't do it without all the support that I have from all the people in the IT. So it's teamwork. So it's not only me.
So I want to do the presentation today about-- and as Cynthia just mentioned I've gone through-- I was so excited about all the projects that we do at the IT. So I felt, Oh, I need to do this, and I want to be involved in this. And that's more exciting. And so if I was giving the choice to play with all the projects that I was interested to play with, and it's amazing. So and that's why I cannot go into details of any of the projects.
But I'll just give you a total overview of each one of them, their idea, and what we do in each project. And I'll answer specific questions if you have them in the Q&A, or maybe if you are more interested in to do the specific details, maybe we could take this offline by email or something. But given the time limits, I cannot go into details of all. But I hope that this presentation will be exciting for you as it is exciting for me to talk about those projects, and it's going to be short and sweet. So if you're not totally related to this area, at least you have a touch of what we do.
So to start with what is-- social robots are different from the normal robots. They both do automated things. They understand their environment and act based on that, but social robots have more-- they should be understanding the social cues from people and they should be suppressing those social cues as well, and have an interaction with people. That allows them to be different, and that allow them to be social.
And because they're social robots, they will expecting different things from them. And they should have a specific unique characteristics that allows them to be what we expect them to be. For example, the embodiment allows them to express emotions, excitement or sadness. Or through their body movement or their facial expression even they're special, but they should be able to do that through the embodiment.
But once these emotions are expressed through embodiment that allows us as people to see them as entities that's not tools. Just something like your pet or something like you had a relationship with, because you don't see it as an object anymore. And when you create a relationship with the social robots and you create a report, that allows the robot to have more impact into your life. Could help you to change your behaviors, or assist you with things that you might neglect otherwise, which we will talk about in second project that we'll go through.
Social robots, and I think this is one of the great things I love about Jibo, it's proactiveness. So Alexa for example, she would not answer you, unless you ask her something. But Jibo, which for example when I wake up in the morning, and go "Hey, good morning. How did you sleep?" And it's great relationship. You feel the emotions, even though you know it's artificial intelligence, it's a tool. But still you still keep having this attachment to it because of this proactiveness. And of course, with Jibo and other social robots they should be able to show emotions and understand emotions in order to be able to reflect or interact with it properly. So that's what makes the social robots different than the normal robots.
And we have the pleasure to have Jibo in our lab, and it was initiated by Cynthia. And the design as you could see, I mean, when I go to the lab and I see Jibo's ancestors, how Jibo have been developed based on all the experience of the other robots, and how it's being shaped the way it is, it shows you how much effort has been done to design it. And so it still hurts me that Jibo is not commercialized anymore.
But we had the opportunity to have it, and we have the SDK to expand on this, doesn't really have as commercial Jibo. And that's something that is amazing and very unique about our group. So the main work that we do is using Jibo as the social robot, and that now I'm taking you through the projects that maybe I'm involved in. So one of the-- so maybe you already know and Cynthia has already mentioned that my background is in effective computing and understanding human behaviors and how can we interfere these behaviors to understand what person mood or their actions or so.
And when I applied to IBK-- and I think I want to move forward. I want to use this technology of understanding humans in an intervention. And when I saw these social robots, realized that that's it. I'm not going anywhere but here. So it was my first choice to use the tools that I have into an entity that can use them to interact with people. So I was so blessed and I was so anxious, if they didn't accept me I'd be devastated.
But I was so blessed and I'm very happy when I got accepted to join the group, and that was one of the first projects that I worked on when we have Jibo to interact and intervene as a coach for people to have positive psychology sessions. For example, it's not for depression, it's not for a specific illness, but it's just for our own mental health. It takes you through sessions like savoring, it would ask you, oh today, I want you when you go out, if the wind breeze in your face, just enjoy the moment. Enjoy the breeze.
And just because these things we don't usually pay attention to in normal life, so when Jibo reminds you of these things and you go out and feel, oh it is nice.
So you start appreciating the small things that happens in your life that we usually go through quickly. So we created this extra skill, Jibo lives in people's homes, and we implemented this into the other skills it has. And as you can see in the picture, you have the station where the extra resources are shown in the tablet, and then there is a raspberry pie inside this thing that also have more of the analysis of the voices and such things.
And then take the interaction with that. So in the first phase we did only seven sessions where the robot lived an average of 10 days in people's homes and now we're doing the second session where the robot lives in people's homes. So it's a two month study, but Jibo lives in people's homes for one month. 14 sessions.
So we want to see how the long term effect, if it has any effect. So because this is one of the things that went, when we did the first session, we had very significant improvement in people's moods. So we thought, oh, is it like the novelty effect because it's just short, or would it affect more. So that's what we're doing with the longer term, to validate our finding from the first one.
I'll show you a quick video to see what I mean.
SPEAKER 1: How is your Thursday going?
SPEAKER 2: Coming, coming, coming. Oh. My day is going well, despite how it might look. Yeah.
SPEAKER 1: I really appreciate you sharing that with me.
SPEAKER 2: Yay.
SPEAKER 1: I'm so curious, did you have the chance to deliver your gratitude letter?
SPEAKER 2: I did, actually. It was pretty nice reconnecting.
SPEAKER 1: Today we are going to do a savoring exercise.
SPEAKER 2: All right.
SPEAKER 1: Do you know what savoring is?
SPEAKER 2: I'm not sure.
SPEAKER 1: I see.
SPEAKER 2: I think its preserving the taste something?
SPEAKER 1: Savoring means fully feeling, enjoying, and extending our positive experiences. It's a great way to develop a long lasting stream of positive thoughts and emotions because we can't always rely on positive events to make you happier. Savoring helps us enjoy experiences that we normally hurry through.
SHARIFA ALGHOWINEM: So I hope that you heard the voice. So that's the idea, it takes you through it and it's only like very short interactions like three to five minutes. And then the next day will ask you, oh, what did you do with the savouring, how did you feel?
So it asked you for reflections of the [INAUDIBLE]. The second project, and it was I was so excited about this too. And one of the things that I loved in my previous work is to have sensors integrated to what we do.
So we worked on sensors like the smartwatch and just sending your emotions or your movement and so on. But with Jibo, I really wanted also to have Jibo have this capabilities of reading other sensors so it will have more expanding of his environment.
So one of the projects is with the medication adherence. And when we say medication adherence, we're not talking about reminders. It's we have mobile app reminders, and we have a lot of other tools to do that. But it's not only that people forget to take their medication, there are more dimensions into it. Sometimes it's just they don't like the side effects, or against their beliefs or they feel its benefit doesn't overweigh the side effects or the cost.
So there's too many dimensions to it, and we're thinking if the robot knows or have the ability to track their medication intake, maybe it could provide more of a dialogue for them to express. Because sometimes they don't want to express themselves to their doctor because of shame or stigma or whatever. So if we provide them with a tool that is able to start a dialogue to see why they're not taking their medication or how the robot can help them in their medication intake, we thought that would be something that a robot could do that might-- and it's affordable and accessible compared to face to face interaction with doctors.
So what we did was we collaborated with [INAUDIBLE] groups for them to have-- so we have each pill bottle would be attached to our NF ID tracking system, and the sensors would be reading the sensing and even I could see when the bottle is lift up or down. And this real time stream is being analyzed and sent to Jibo, so Jibo could interact to say, oh, this is proactively for example, it's not the time for medication, or you already have taken your medication or such things. And that would allow Jibo to help on, for example, Alzheimer people when they forget that they took the medication and they take it twice. And that help Jibo to help them not to overdose, for example, or such things.
I'll be showing you a video that is-- I'm focusing on proactiveness. So in the individual, I'm taking the medication not on the right time. And this is what Jibo says to me.
[START VIDEO PLAYBACK]
- Please don't take Lipitor now. You've already missed a dose. It is best to wait for the next dose, which will be in 13 hours. I will remind you then. Is that OK with you?
- Oh, can't I have it now and again later?
- According to the instruction, it's best to wait for the next scheduled time. Don't worry, doctors say missing a dose occasionally is all right as long as you start taking it again at the right schedule. I'll make sure to remind you at the right time. I'm here for you.
[END PLAYBACK]
I love this project very much because it allows us to see how the sensors could be integrated and used and read by Jibo to start the intervention. And that allows it to have more accessibility to our environment, and then we'll be able to assess us. So if for example, if we are able hopefully, one day we'll be able to connect Jibo with our smartwatch, and then we'll say, oh, you're not moving for three hours. Maybe just stand up and sit down, and so just to help us with our health, our movement. And I think it will open up a lot of other helpful ways for Jibo to help us in our daily life.
So we're scaling up now. We have one on one interaction, and now we're having dual interaction. So we have this project when we want Jibo to be a part of the family environment to help parents in their daily activities with their children. If they're reading a story, how can Jibo interact in a way that illicit and nudge and encourage critical thinking.
So let's say the mother is only reading or the parent is only reading without asking any questions, when Jibo enters and say, oh, why the frog do like this? Or why this person's wearing shoes that is bigger than him? Or something like this that allow the child to question, and then able to think and critically analyze the environment around them, if it's a book. So just an encouragement for them to have a better learning experience and a better development of their critical thinking.
So this project is divided into three phases. So phase one, just we want to understand how the parent and the child read stories in a normal way without a robot. So we had them reading a story from a tablet, and then we analyzed-- we had to track their bodies. Let's find the joints, triangulate it to a 3D thing. And we wanted to see what's their behaviors, and how can Jibo know this style of interaction between the parent and the child.
So some parents would allow the child to do the whole reading, and then some others will do the reading. And then some children will be totally annoyed or bored, and then it will be just moving a lot. So I think understanding this movement-- based on our analysis, we were able to detect the frustration of the parents when the child, for example, was not paying attention or totally distracted by the environment around them.
And you could see the frustration in the parents, and you can also see the boredom in the child based on their behavior. So knowing those signals, can we adjust the behaviors of the robot? Can we make the robot, for example, more-- when the parent is frustrated can the robot intervene to the child, so it could just grab their attention, for example, or do something like that?
So this is one way for Jibo to understand the behaviors of the parents. We were able to predict what the style of parenting in there, so you could see from the-- for example, parents who are used to do asking questions and being interactive and doing more reading at home, they have certain behaviors. And even the child have adjusted to those behaviors, and you could see that he's engaged. Children who are more doing more story reading and more interactive story reading are more engaged based on their behaviors only. So it feels like we are opening up an avenue for the robot to have more understanding of the relationship between the parent and the child, and therefore being able to personalize the intervention between them.
So the second phase-- I thought we could go to the next slide somehow. Yeah, so the next phase is we want wanted-- so we now understand the behaviors. Can we understand when the robot should intervene? Because in the previous study, we did not have a robot, so we don't know when the robot should.
How can we calculate when the robot should intervene or start the interaction? Because you don't want the robot to interrupt the mother when she's reading. Or you don't want it to be seems like invading the space of the child and the parent if they're discussing something. So what we are doing now, we're created ulterior operated system. So this station is being deployed in some people's homes, and they will be reading stories of the tablets.
And then Jibo will be asking questions based on the content of the story. And this content has been automatically generated. Some of them are manually generated. And then the ulterior operator will choose one of these questions based on the timing and the page of the story that they're on and so on. And then once we finish this, we want to analyze what's the best time, and what's the perceived relationship with the robot when it's there.
Do the parent and the child feel that they're invading their space? Or do they feel that they're totally integrated and smoothly interacting with them? So that once we analyze this, we'll be able to do the third phase when we're totally automating the whole thing, behaviors and timing and choosing the question and all of this to have to Jibo then interacting in a family environment in a smooth way.
This project is just-- I did it just for fun. I couldn't feel good if I leave PRG without making Jibo speak Arabic. So I felt that's just not going to be offensive.
So Matthew Huggins created a language model that understand intent based on a very small sample size, very small number of statements. So the threshold was only 25 statements per class. And that was to me, I was like, OK, this is an opportunity. I could create something that it's easy that I could just brainstorm or create something quick in Arabic. So we did that.
And then we challenged ourselves to do it in a different other languages, so we did it Korean and Spanish and Arabic. And this is just a small interaction to show that we can create something that is useful for other languages. But you will notice that one of the bad things that I really hated about this is that Jibo voice has been designed carefully to be the way it is.
But when we change the language, you cannot use his voice, and therefore it becomes awkward. So just bear with it. Maybe we could find a way to make this voice as he is, even in different languages. But I'll just jump through. Because it's a three minute video I'll just jump through, so we could see the intent recognition and the interaction.
[START VIDEO PLAYBACK]
[SPEAKING KOREAN]
[SPEAKING SPANISH]
[SPEAKING ARABIC]
[END PLAYBACK]
So it's a bit slow because it takes the statement, translate it, create the audio file, and then send it back to Jibo and Jibo speaks it. So that's why it's slower than usual. But the idea is we were able to create a model that is in a different language that can understand and can have a dialogue with Jibo automatically.
So all of this is automated, even the generation of the audio. That's why you could see the lag because it's just the audio is created, then played in Jibo. If we were able to somehow use Jibo's voice, that would be automatic. Well, I think it would be faster because it's just text to speech instead of creating a wave file and play the wave file. But it's an opportunity that we could see and we could play with, but that was just for fun, so I'll be back to serious now.
One of the things that we also did and that is not including the robots. The projects that I've mentioned, we're using the robots. But those were just maybe a bit for how to use the robot on these. So two projects that is on this.
One of them-- now with the emergence of deep learning models, they act as a black box. We don't know what's going on. We don't know what they're looking at. We don't know what the patterns that they become. And when we talk about depression and suicide and all of these, we are talking with psychologist, and I don't think they can trust a model that gives you 100% accuracy in diagnosis that's better than them without telling them what's the model was looking at.
So current methods in deep learning explanations are on image only. So they do heat maps, they do-- but there is a very limited and almost none that acts with videos. So when someone is moving, I cannot see from one image that someone is depressed. But I could see from like two minutes into action, I might be able to see that they are acting with depression or their emotional expressions are not aligned with their mental health or what we expect.
So what we did, we create something that is-- we scaled up the methods that is applied for images to have them on videos. Not only this, most of the explanation methods in deep learning are quite qualitative. So a human observation has to look at them and see what are they looking at.
We wanted to automatically quantify, create something that we can quantify automatically to see what is the model was looking at without human observations because we have thousands of sample points. We can not look at each one of them and see what the model is looking at. And if we cherry pick, that would not give us enough information.
So what we did, we used not only heat maps, we used attributions of the models and the weights to see what the model's looking at in what context. And we divided this context in two things, either regions to see, OK, what's the difference between the eyes of the depressed person and non depressed person? What's the difference in the mouth region? What's the difference in their facial regions and so on. And you could see from the depressed people, you could see there is the attention is more into their forehead and nose, and I'll show you why now.
And then with the minimum depressed or non depressed, the attention is more into their head movement, as you can see, and maybe eye movement. We don't know. So that's the attention we think of that it might be the case. So when we divide the regions, we could see that there is more attribution to the nose of the depressed person, while it's the more attributions to the mouth in the non depressed person.
Then we said, we don't know enough. A region is not enough. Maybe we could do more. So we did the action units.
Action units are the specific muscles that once activated, it shows you what emotions are we expressing. For example, if those two muscles are activated, you're smiling. If those muscles are activated, you're angry and so on. So it's just muscles. That combination of them will show you what emotions are being expressed.
So when we did the correlation between the attributions and the action units in those video frames, you could see anyway from the image-- it's too many action units anyway-- there is less activation in the depressed people, which is aligned with the literature. They don't show too much of activations of their face muscles compared to less depressed. They would have more movement or more emotions, and they're expressing more emotions.
And looking at this, we found that the action units that is activating disgust, which is in line with the literature. Depressed people express more disgust than normal. And the disgust will always goes into the nose region and the forehead region. And if they're talking with disgust, that those muscles will be activated, and therefore we can say that our model looks at the right things by looking at those specific actions or emotions that the depressed person is expressing. So we were able to provide a video based deep learning analysis and explanation model that shows that is assigned and associated with the regions and the action units of the person.
The second project on this aspect is we had a collaboration with Japan Broadcasting Corporation. And they provided us with a-- so they have a big program on suicide intervention and awareness. And it's a big problem in Japan, especially in youth, like 16 and above. There's a lot of-- it's very sad.
So they do a lot of programs to allow those young people to know that they're not alone, and that they can have support and so on. So we collaborated with them to get the deficit to see can we detect or see depression or suicide behavior while we are just naive observers waiting in the bus. So just to analyze what is the behaviors that can indicate their level of suicide risk.
In this project, we have human observations to look at with other depression behaviors, anxiety behaviors, and physical disengagement and so on. So manually annotated and analyzed. And we hand crafted features, which tracked how many times the blinked, how much frequency of the head movement.
Do they look down? Do they look up? And those handcrafted features that we carefully select based on the literature. And then we also have the deep learning models when we say, OK, we use a black box. We don't know what does it say or what does it see and what does it here, but can it give us enough information about the detection about the suicide risk level, and this is still in the go.
The last project where we scale up more-- so we did one on one robot interaction. We did two, double, where we have to try the interaction. And now we want to scale up to a multi-party robot interaction.
We have this project when we want to have Jibo in a museum context. And when it's in a museum, you want to know those four or five people are a group, and then you need to identify the group. And then you have to understand each one person behaviors, and then how their behaviors integrate together to see the dynamics of the group.
So who is the leader of the group? Or are they happy? Can Jibo say, oh, I suggest that you go there? Or are they frightened that Jibo should not talk?
Or maybe look at their-- I mean, and also once Jibo started the conversation with them, it should know their preferences. And it will say, oh, I think the third floor there's something "blah, blah" that you might interest you based on the conversation that he had with them. So they would have a better experience in the museum.
So those are the projects that I am involved in. And I'm always excited about getting involved in more exciting projects. But sometimes the time doesn't permit.
But beyond the projects, of course, we did a lot of trainings, teaching certificates, where there are several workshops at MIT of leadership and entrepreneurship and patents and all of these beautiful resources that MIT provides. And that was before the pandemic. After the pandemic I am enough with Zoom meetings. I think I can't wait until it's opened again, so we could do in-person workshops too.
I supervised the master of engineering student that we worked on the depression expression behaviors, as I've mentioned before. It was a great experience to give my student a place to be creative while still accomplishing their work. And also I supervised a lot of UROP students. And I've confessed, in the last year I had six UROPs in my plate, and all of them were freshmen.
Reaching this stage, I feel so awarded and so accomplished looking at them starting from freshmen like not always clean slate there. A lot of them have a lot of experience already. But going from there to see them, like last week one of my students submitted a paper at a conference, and I felt so proud of that paper, more than I'm proud of my own papers. It just feels like you feel the reward, that you took someone from somewhere, you put them to a path.
That is very rewarding for me. I'm sure that she's so proud of herself now. But it's really rewarding to see your efforts as it's being accomplished.
The last thing that I really want to talk about is it's the work environment that we have at PRG. So it's creative, it's dynamic, there's very exciting projects, but also supportive and collaborative and caring. And when you feel down, a lot of people will pick you up. And I never thought this environment would exist beyond the walls of my family and with my sisters.
And I felt if this thing can be created outside the family environment, then I think this is what we should aspire to. And we should have that work environment being applied everywhere else. We should not live in an environment that's less than this. Because it will help in reducing anxiety, stress.
You would wake up because you go to work because you want to go to work. You will go out of bed because you're excited to do work, not the opposite. And I think that is what we should aspire. If the world or the work environment looks like this, I don't think we'll have mental illness.
And that's all for me. And I hope that I wasn't too long. And I hope that it was still exciting without giving you too much of boredom.
DOROTHY HANNA: That was just right, Sharifa. Thank you so much. Yes.
And I can see with the exciting work you're doing why you could create a really compelling work environment like that to match with the work you're doing to make life better for other people. So I'm going to open it up to questions. If anybody who's joined us today wants to ask a question, put it in the Q&A. But while people are thinking of questions, I don't know if Professor Kamal or Professor Cynthia, if you have anything to ask Sharifa?
CYNTHIA BREAZEAL: Sure, maybe I'll just start. And again, I think you can see why Sharifa has been such an important member of our team and has contributed and really just fit into the group and the group culture just so seamlessly and in so many ways. And she's become, again, this beloved, important, cherished person in the group.
So and Sharifa clearly is very prolific. She's done so much, worked on a number of different projects, has really been there helping a variety of students with her expertise, as well as learning from the group as well. So I guess just at a high level, Sharifa, I guess I'm just curious, like in terms of what you feel like has benefited you the most career wise, what experience do you think has done that for you the most over the time you've been in the group?
SHARIFA ALGHOWINEM: I think everybody-- looking at me before the group and now, there is a lot of technical stuff that I have learned and expand. Looking at interaction to begin with, my work was always only just detection, and now moving forward to the interaction, that is a different style. So even how Jibo should say things and what's the movement that comes with it. That is totally different than my background.
So really I felt how much of the design that is need to be there and how even the dialogue design is not than just straightforward. It's not like writing. It's totally different, it's different dynamic.
And then moving to having two people, and then more than two people. I felt was something I have never worked on. From the learning curve, it's really moved me a lot, like critically my learning curve gone wildly.
But also the emotional aspect, the environment I feel this is what I felt that we were missing in normal life, that we don't have anywhere else. I think that's feel if-- I don't want to leave-- but whenever I leave, I will aspire to create an environment for my student or for my colleagues that should make them feel supported and happy and waking up knowing that they're coming in a safe space. I think that's the thing that I feel it's very important beyond the work.
THERESA WERTH: Right, as I unmute, I thought there was a big sound. I was curious just to that, but, Sharifa, have you thought about how you might incorporate this technology into the workplace towards that goal? I liked a lot what you were sharing about using social robots and families. But in a workplace, you spend a lot of time together. It seems like there's a commonality there.
SHARIFA ALGHOWINEM: Yeah, I think that needs a lot of research and a lot of brainstorming with Cynthia and how did she manage to create this culture and how it's being enforced by all the people. So we have Polly as our cheerleader, and then we Hae Won, who enforce the culture. So we need to get brainstorming session with them on how to integrate that to a social robot that could also keep enforcing this.
THERESA WERTH: OK thank you.
KAMAL YOUCEF-TOUMI: Yeah, Sharifa, for the presentation and all these exciting projects that are happening in Cynthia's lab. I was thinking maybe for the children because sometimes they may need motivation, or I think you mentioned some of this in your presentation, not only in-- what is it the level of the robot to start with? And then at interacting with, let's say, children of a certain age, then the robot itself can be build more of whether its the experience or knowledge, so that it can raise in, for example, understanding or activities that it's doing well with children.
SHARIFA ALGHOWINEM: And so I talked about only the project I worked on. Of course, PRG is way much bigger, and they have a bigger aspect when they're looking at children education, and then AI and how they create activities for the children for reading and for AI and how to interact with tools. And it's way bigger than me because I'm not educational person. So I think maybe Cynthia could help out with a few sentences about that to answer your question.
CYNTHIA BREAZEAL: So can you reframe the question, again, to make sure that I'm answering the right?
KAMAL YOUCEF-TOUMI: Sure. The thing that if these types of robots are, let's say, targeting the young children, right. So what would be the level of understanding or information that the robot will have on one hand? And then as it is interacting with, let's say, a child or different children, then the robot would be improving and adding to its knowledge, so that when the robot is interacting with the children, every time it's raising the level, challenging them and so on.
CYNTHIA BREAZEAL: Yeah, so we have a whole other line of research within the group are on adaptive personalization. So as the robot interacts with individual children over time because these are long term engagements, so the robot keeps interacting with the same child over and over as they play a variety of educational games with the robot. So Hae Won Park, our research scientist, she does a lot of this work in applying reinforcement learning to learn a policy of personalized policy for each child in terms of not only kind of how to adapt the curriculum, but how to actually adapt the robot's behavior as well.
We have another PhD student looking at cross task training, so all of these games fit together within a larger curriculum. So they're reinforcing concepts in different ways and skills in different ways. And so we're looking at ways that rather than having a personalized policy for each particular activity separately, the more it gets experience playing a certain kind of game, can that help accelerate the personalization across a different but related game.
So we're looking at a number of different dimensions in terms of how you do this adaptive personalization over time. But our group is among the first, I think, to actually show that doing this with young children in real preschool and kindergarten classrooms. So in a lot of ways, we're leading the field for early childhood. We've been really focusing on early childhood as a critical time to intervene.
There are certainly people who have worked in more STEM related fields for older students in things like math and science, but learning literacy skills for young children is quite different because a lot of it is done through social interaction, and a lot of it is very playful. And in fact, when you think about children's books, this is an area that we're just trying to develop more. There's a lot of common sense understanding that's assumed, especially the younger the books that children are reading, and common sense is one of the hardest areas of artificial intelligence.
We're kind of good at the specific, encyclopedic, factual kind of knowledge, but this underlying understanding of how the world works around you, that's what's really hard for AI. But that's what children are coming in with, all right, so it's pushing our research in new directions when we think about the intersection of common sense and dialogue and interaction, which is actually really exciting. So anyway, yeah, the adaptive personalization, it is a really important area.
KAMAL YOUCEF-TOUMI: Thank you, thank you. I think this is very exciting. So Sharifa, when you go back, are you going to replicate Cynthia's lab somewhere?
SHARIFA ALGHOWINEM: We don't have Jibo. And I'm not an engineer to create a new robot. But I think we could borrow a few of the things that we learned from the lab and see how we could customize it to the resources that we have there.
BARBRA WILLIAMS: Now is Jibo an acronym?
CYNTHIA BREAZEAL: No, the name of the robot.
DOROTHY HANNA: I had a question about teaching Jibo to differentiate between the parent and the child and figuring out who was frustrated and everything. How did that go? How did Jibo understand who was the adult in the relationship? I was fascinated by the vectors on the screen and stuff. But whatever, if you could say more about that?
SHARIFA ALGHOWINEM: Well, so it's a big problem. It's a very big problem in the AI environment. So it will take me a while, but I'll try to just slice it down.
DOROTHY HANNA: Thank you.
SHARIFA ALGHOWINEM: So there is a problem of detection. So in each image, you find a person. And then you have the problem of tracking. You want to keep the same person, the one that you detected in the first frame to be tracked in several frames, even if they switch places, if they move, if they're cover their faces with a book, and then come back, or they hide behind their parents or whatever. So it's the [INAUDIBLE] problems that could hinder our checking algorithms.
So looking at the literature of airport surveillance, when they tried to find the person from one frame or even camera view another camera view-- and this person could be obscured by walls or other people, and this person could carry a bag, and then the bag could be in the other hand, so we cannot rely on the appearance of that object, but we have to rely on what segments of that object that exists. So in the airport surveillance, they do patches, like they take part of the face, part of the shoulders, part of the bags and so on, and they keep fact checking those small patterns to see if they still exist and therefore track the person. And then you come up-- so this is how the advanced tracking happens, and which I have adopted in this segment, so to identify the person and keep tracking them.
And now we have the problem of who is who, as you have just mentioned. And then I added to that the face recognition. So if we could have the face imaging and train it on, oh, that's a parent or person with the age of such and female or male or whatever, and that's their face. And you keep tracking. Once you track the bodies, you want to track the same face.
And then you keep knowing that this is the adult or, as you say, based on the age or gender or size, and then that's the child. And that's where the tracking and the identification happens.
So it's three layers, detection tracking, surveillance tracking, then face recognition. It's a very long answer. But I was so excited about this because it took me three weeks to just manage how to make it happen accurately. And because we know that this is going to be deployed in people's homes, and we want it to work without any manual limitation, and therefore I had to spend a good amount of time to just nail it, and I did.
DOROTHY HANNA: Good job. So then at the same time, you're also tracking their emotional state to some degree?
SHARIFA ALGHOWINEM: So I'm not a fan of facial expressions, even though I know how great they are. It just the tools that we have now they're trying to respond in acted emotions. So I am happy now, but I'm not smiling, and therefore the detection will not tell that I'm happy because it doesn't know the subtle, spontaneous facial expressions because it's been trained on actors or from movies.
And they should exaggerate their emotions to show you what you wanted to learn, and that doesn't work in everyday life. So I feel strongly more about the body behaviors and the head movement and eye gaze because I think there we can detect them automatically, and then we could have an inference of what they mean with the other queues. So not facial expression, but more of the how their head is moving, are they looking at the book, are they looking outside, are they looking at their mothers or the parent's faces?
And that will help me a lot on what to understand where they're at. Are they bored? And then for example, their body orientation. Are their body orientation is to each other or against each other?
And even from the suicide, we found that the head movement and eye movement and blinking is a bigger cue for identifying the person who is highly suicidal than someone with a lower risk of suicide when they're not moving enough, or they're looking down too much, or they're not having too much of variance of their movement. Because it's spontaneous and we can detect it automatically I think it's more powerful.
DOROTHY HANNA: Really fascinating, thank you. We're coming up on the time. Any other questions before we need to wrap this up?
KAMAL YOUCEF-TOUMI: No. I just want to thank Cynthia for, as I said earlier, not only hosting Sharifa, but involving her and supervising her closely with the work. And we thank you very much for that, Cynthia. And Sharifa, congratulations on your good work.
SHARIFA ALGHOWINEM: Thank you.
KAMAL YOUCEF-TOUMI: Yeah, and I'm sure that you will go on and do a lot great things beyond MIT.
SHARIFA ALGHOWINEM: Appreciate it, thank you. Yeah. But I also thank Cynthia for hosting.
To me it was a long shot, but I was so grateful, and I'm still grateful. It's a great environment and great, exciting projects. And I'm grateful for them to be patient with me when I struggle a few things.
CYNTHIA BREAZEAL: It's just been such a delight having Sharifa in the group. So we were very lucky to have you, Sharifa.
SHARIFA ALGHOWINEM: Thank you. That's embarrassing, but thank you.
DOROTHY HANNA: Well, Sharifa, thank you so much. It's great to hear about your research. And yeah, thank you to everybody for being here today. And have a great rest of your day.
SHARIFA ALGHOWINEM: Thank you.
CYNTHIA BREAZEAL: Great, thank you.